CANTEMIST (CANcer TExt Mining Shared Task – tumor named entity recognition)
The CANTEMIST Track is sponsored by Plan de Impulso de las Tecnologías del Lenguaje (Plan TL)
Generated resources
- Data: Gold Standard, Silver Standard & Annotation Guidelines
- Conference Proceedings
- YouTube presentation
- Participant codes
Please, cite us:
Antonio Miranda-Escalada, Eulàlia Farré-Maduell, Martin Krallinger. Named Entity Recognition, Concept Normalization and Clinical Coding: Overview of the Cantemist Track for Cancer Text Mining in Spanish, Corpus, Guidelines, Methods and Results. Proceedings of the Iberian Languages Evaluation Forum (IberLEF 2020), CEUR Workshop Proceedings. 303-323 (2020).
@inproceedings{miranda2020named, title={Named entity recognition, concept normalization and clinical coding: Overview of the cantemist track for cancer text mining in spanish, corpus, guidelines, methods and results}, author={Miranda-Escalada, A and Farr{\'e}, E and Krallinger, M}, booktitle={Proceedings of the Iberian Languages Evaluation Forum (IberLEF 2020), CEUR Workshop Proceedings}, year={2020} }
Background and motivation
There is a pressing need to apply natural language processing (NLP) and text mining technologies to process clinical texts in order to unlock critical information that enables better clinical decision-making. NLP can facilitate the use of information from literature and electronic health record in biomedical data analysis. Understanding diseases requires the extraction of certain key entities like diseases, treatments or symptoms and their attributes from textual data, as has become clear from the recent COVID-19 (SARS-CoV-2, coronavirus disease) pandemic, which showed the current struggle in processing clinical documents written in various languages.
With over 470 million native speakers, there is a worldwide interest in processing medical texts in Spanish (every 10 minutes, tens of thousands of EHRs are produced just in Spain). Such technologies have also the potential of being adapted to handle other languages, like Italian, German, French or even English.
Results of systems capable of automatically processing clinical texts are not only of interest for the medical user community or researchers working on basic and applied health-related disciplines, but are also demanded by the pharmaceutical industry and ultimately by patients.
Due to the special relevance of cancer as one of the leading causes of death and the growing healthcare expenditures for oncological treatments a specific classification resource for oncology has been constructed by the WHO known as International Classification of Diseases for Oncology (ICD-O) or as eCIE-O-3.1 [1] in Spanish. This resource characterizes the histological type of neoplasms, known as morphology (morfología de neoplasias). The CIE-O has been used for over 25 years as a standard resource to code diagnosis of neoplasms in tumor and cancer registries as well as pathology reports [2].
The use of NLP techniques may result in applications that could contribute to both oncological and clinical research and potentially collect important clinical variables for cancer treatments hidden in medical texts, which are key to foster health quality improvements and personalized medicine in a more systematized way for advancing cancer care.
Task summary
Following the success of previous shared tasks we have coordinated in collaboration with the BioCreative challenges (e.g. ChemDNER, ChemProt), BioNLP-OST (PharmaCoNER), eHealth CLEF (CodiEsp) or IberLEF2019 (MEDDOCAN) we are organizing the first shared task specifically focusing on named entity recognition of a critical type of concept related to cancer, namely tumor morphology, called CANTEMIST. These previous efforts results in high impact datasets, publications and new tools.
Due to its practical relevance, clinical records are being indexed or ‘coded’ with standardized tumor morphology concepts in many countries worldwide using the ICD-O codes (International Classification of Diseases for Oncology, 3rd Edition – ICD-O-3).
Following this demand on tools to extract tumor morphologies, the CANTEMIST track relies on a manually annotated corpus generated by experts in oncology with the aim of automatically detecting mentions of tumor morphology terms as well as linking them to their corresponding ICD-O codes.
Task description.
CANTEMIST will explore the automatic assignment of eCIE-O-3.1 codes (Morfología neoplasia) to health-related documents in Spanish language.
The CANTEMIST task will be structured into three independent sub-tasks, each taking into account a particular important use case scenario:
- CANTEMIST-NER track: requires finding automatically tumor morphology mentions. All tumor morphology mentions are defined by their corresponding character offsets in UTF-8 plain text medical documents. Example (the start and the end character offsets are highlighted in bold):
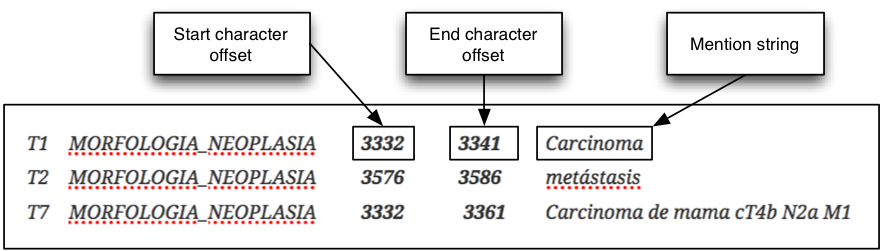
- CANTEMIST-NORM track: clinical concept normalization or named entity normalization task that requires to return all tumor morphology entity mentions together with their corresponding eCIE-O-3.1codes i.e. finding and normalizing tumor morphology mentions. Example (the ICD-O-3 codes are highlighted in bold):
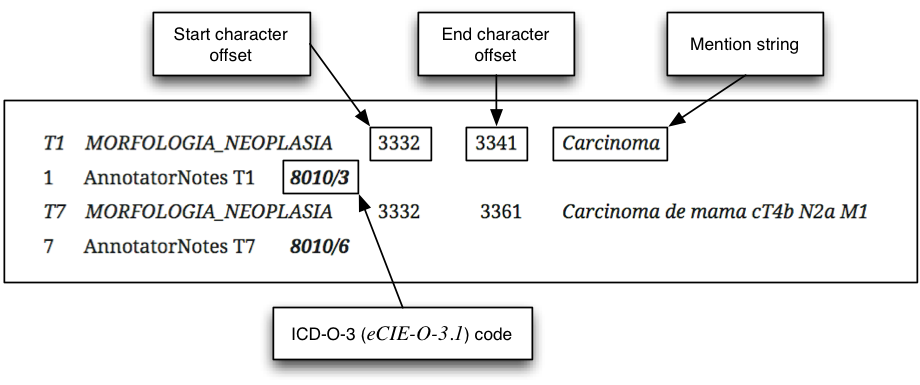
- CANTEMIST-CODING track: requires returning for each of document a ranked list of its corresponding ICD-O-3 codes (Spanish version: eCIE-O-3.1). This it is essentially a sort of indexing or multi-label classification task or oncology clinical coding. Example (the ICD-O-3 codes are highlighted in bold):
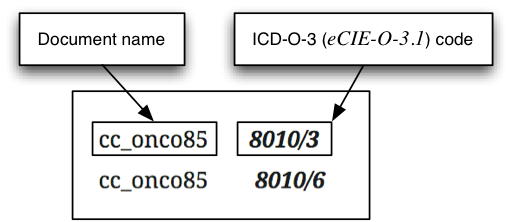
CANTEMIST Gold Standard corpus
Participation at the CANTEMIST track allows access to a manually annotated Gold Standard corpus generated by experts in oncology. Quality of the dataset was assured through elaborate annotation guidelines and consistency analysis (inter annotation agreement). The clinical cases used for the CANTEMIST corpus were manually selected and carefully per-processed, in order to resemble the content of oncology clinical records covering a diversity of different cancer types, both common as well as rare cancer cases.
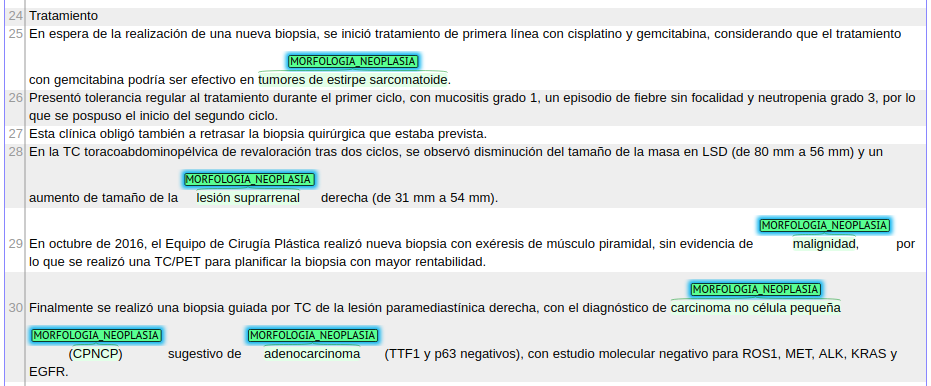
[1] Clasificación Internacional de Enfermedades para Oncología (CIE-O) – 3ª edición, 1ª revisión
[2] https://eciemaps.mscbs.gob.es/ecieMaps/browser/index_o_3.html
Overview Video
Selected References
[1] Seda, Silvia Sánchez, et al. “Plataforma para la extracción automática y codificación de conceptos dentro del ámbito de la Oncohematología (Proyecto COCO).” Procesamiento del Lenguaje Natural 61 (2018): 65-71.
[2] Kavuluru, Ramakanth, et al. “Automatic extraction of ICD-O-3 primary sites from cancer pathology reports.” AMIA Summits on Translational Science Proceedings 2013 (2013): 112.
[3] Coden, Anni, et al. “Automatically extracting cancer disease characteristics from pathology reports into a Disease Knowledge Representation Model.” Journal of biomedical informatics 42.5 (2009): 937-949.
[4] Martinez D, Li Y. Information extraction from pathology reports in a hospital setting. InProceedings of the 20th ACM international conference on Information and knowledge management 2011 Oct 24 (pp. 1877-1882).
[5] Bozkurt, Selen, et al. “Using automatically extracted information from mammography reports for decision-support.” Journal of biomedical informatics 62 (2016): 224-231.
[6] Qiu, John X., et al. “Deep learning for automated extraction of primary sites from cancer pathology reports.” IEEE journal of biomedical and health informatics 22.1 (2017): 244-251.
[7] Oleynik, Michel, Diogo FC Patrão, and Marcelo Finger. “Automated Classification of Semi-Structured Pathology Reports into ICD-O Using SVM in Portuguese.” Studies in Health Technology and Informatics 235 (2017): 256-260.
[8] Deshmukh PR, Phalnikar R. TNM Cancer Stage Detection from Unstructured Pathology Reports of Breast Cancer Patients. InProceeding of International Conference on Computational Science and Applications 2020 (pp. 411-418). Springer, Singapore.
[9] Jin, Y., McDonald, R. T., Lerman, K., Mandel, M. A., Carroll, S., Liberman, M. Y., … & White, P. S. (2006). Automated recognition of malignancy mentions in biomedical literature. BMC bioinformatics, 7(1), 492.
[10] Spasić, Irena, et al. “Text mining of cancer-related information: review of current status and future directions.” International journal of medical informatics 83.9 (2014): 605-623.
[11] Si, Yuqi, and Kirk Roberts. “A frame-based NLP system for cancer-related information extraction.” AMIA Annual Symposium Proceedings. Vol. 2018. American Medical Informatics Association, 2018.
[12] Tanenblatt MA, Coden A, Sominsky IL. The ConceptMapper Approach to Named Entity Recognition. InLREC 2010 May (pp. 546-51).
[13] Xie, Boya, et al. “miRCancer: a microRNA–cancer association database constructed by text mining on literature.” Bioinformatics 29.5 (2013): 638-644.
[14] Yoon, Hong-Jun, Arvind Ramanathan, and Georgia Tourassi. “Multi-task deep neural networks for automated extraction of primary site and laterality information from cancer pathology reports.” INNS Conference on Big Data. Springer, Cham, 2016.
[15] Yim, Wen-wai, et al. “Natural language processing in oncology: a review.” JAMA oncology 2.6 (2016): 797-804.