CodiEsp: Clinical Case Coding in Spanish Shared Task (eHealth CLEF 2020)
The CodiEsp Track (eHealth CLEF 2020 – Multilingual Information Extraction) is promoted by Spanish National Plan for the Advancement of Language Technology (Plan de Impulso de las Tecnologías del Lenguaje – Plan TL).
Generated Resources
- Data: Gold Standard, Silver Standard & Annotation Guidelines
- Conference Proceedings
- YouTube presentations
- Participant codes
Please, cite us:
Miranda-Escalada, A., Gonzalez-Agirre, A., Armengol-Estapé, J., Krallinger, M.: Overview of automatic clinical coding: annotations, guidelines, and solutions for non-English clinical cases at CodiEsp track of eHealth CLEF 2020. In: CLEF (Working Notes) (2020)
@inproceedings{miranda2020overview,
title={Overview of automatic clinical coding: annotations, guidelines, and solutions for non-english clinical cases at codiesp track of CLEF eHealth 2020},
author={Miranda-Escalada, Antonio and Gonzalez-Agirre, Aitor and Armengol-Estap{\'e}, Jordi and Krallinger, Martin},
booktitle={Working Notes of Conference and Labs of the Evaluation (CLEF) Forum. CEUR Workshop Proceedings},
year={2020} }
About the task
The CodiEsp Track is part of the CLEF 2020 conference. Participants are expected to register on the CLEF registration webpage and submit the working notes to the conference. Attending the conference is also recommended.
There is an increasing interest in exploiting clinical texts by means of language technologies and text mining approaches. Structured clinical information, in the form of codified clinical records relying on controlled vocabularies such as ICD10 is a key resource for statistical analysis techniques applied to patient data. The results of clinical coding are being used, for instance, as aggregated data to analyze retrospective/prospective aspects of information contained in electronic health records (EHRs).
Clinical coding is a key task consisting of the transformation of medical texts written by clinicians into a structured or coded format using internationally recognized concepts o class codes (numeric or alphanumeric format).
These codes usually describe a patient’s complaint, problem, diagnosis, treatment or reason for seeking medical attention.
This transformation of natural language clinical text into structured data is critical in order to enable subsequent use for clinical care, research, and other purposes such as statistical analysis and decision-making or even re-imbursement.
Clinical coding, originally being used for mortality statistics, is now used to classify all patient hospital episodes and procedures.
Due to the importance of this process, there are now even specialized education programs and professional occupations of persons employed as clinical coders or medical records technicians.
Summary of clinical coding results are importance:
- Use to reflect the pattern of practice of clinicians.
- Provide basis for decision-making processes.
- Standardizing the recording of clinical information.
- Enable statistics, data mining and provide structured information for healthcare and bioinformatics.
- Analyse effectiveness of patient’s care and treatment
- Enable aetiology studies, health trends, epidemiology studies, clinical indicators, and patient selection
The importance of clinical coding has to lead to the organization of challenges and shared tasks to promote automatic clinical coding systems using sophisticated machine learning techniques, such as the following eHealth CLEF lab series: [2018] death reports in the French language were indexed; [2019] German non-technical summaries (NTPs) of animal experiments were codified.
We organize the first community task specifically devoted to the automatic coding of clinical cases in Spanish: the CodiEsp task. Participant systems have to automatically assign ICD10 codes (CIE-10, in Spanish) to clinical case documents, being evaluated against manually generated ICD10 codifications. We foresee that this task will be influential not only in terms of determining the most competitive approaches for this particular data and track but it will serve also to generate to learn how to generate new clinical coding tools for other languages and data collections.
Moreover, to improve systems’ acceptance and usefulness, their results must be understandable and transparent. To that extent, in addition to two traditional code prediction sub-tracks, the CodiEsp track proposes a novel sub-track on Explainable AI. Systems that participate in this sub-track are expected to predict the correct codes and present the reference in the text that supports the code predictions.
In order to carry out all sub-tracks, we have prepared a synthetic corpus of 1000 clinical case studies. The CodiEsp corpus was selected manually by practicing physicians and clinical documentalists and annotated by clinical coding professionals meeting strict quality criteria.
The CodiEsp track contains three sub-tracks (2 main and 1 exploratory):
- CodiEsp Diagnosis Coding main sub-task (CodiEsp-D): will require automatic ICD10-CM [CIE10 Diagnóstico] code assignment. This sub-track evaluates systems that predict ICD10-CM codes (in the Spanish translation, CIE10-Diagnóstico codes). A list of valid codes for this sub-task with their English and Spanish description is downloadable here.
- CodiEsp Procedure Coding main sub-task (CodiEsp-P): will require automatic ICD10-PCS [CIE10 Procedimiento] code assignment. This sub-track evaluates systems that predict ICD10-PCS codes (in the Spanish translation, CIE10-Procedimiento codes). A list of valid codes for this sub-task with their English and Spanish description is downloadable here.
- CodiEsp Explainable AI exploratory sub-task (CodiEsp-X). Systems are required to submit the reference to the predicted codes (both ICD10-CM and ICD10-PCS). The correctness of the provided reference is assessed in this sub-track, in addition to the code prediction.
For this task, we have prepared a corpus of clinical cases. This CodiEsp corpus of 1,000 clinical case studies was selected manually by a practicing physician and annotated by coding professionals.
Below we show an example of a clinical case annotated in the Brat visualization tool.
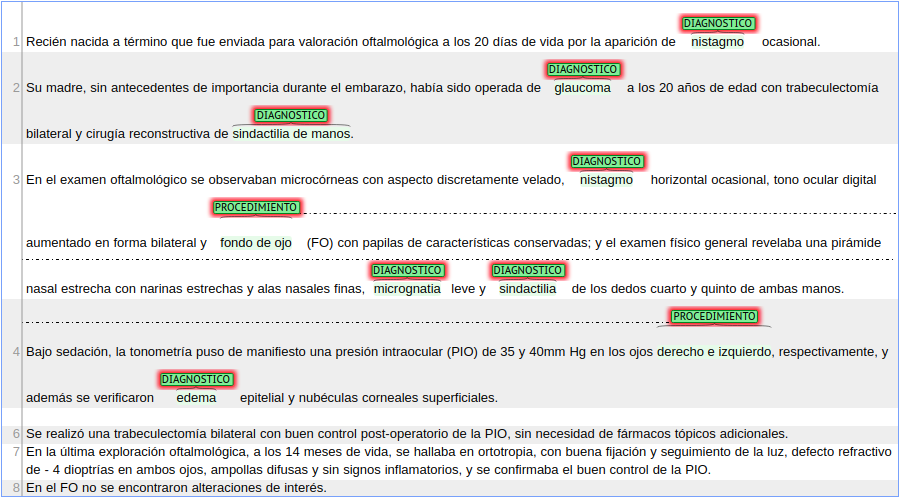
For more detailed information see Description of the Corpus.
References
[1] Stanfill MH, Williams M, Fenton SH, Jenders RA, Hersh WR. A systematic literature review of automated clinical coding and classification systems. Journal of the American Medical Informatics Association. 2010 Nov 1;17(6):646-51.
[2] Xie P, Xing E. A neural architecture for automated icd coding. InProceedings of the 56th Annual Meeting of the Association for Computational Linguistics (Volume 1: Long Papers) 2018 Jul (pp. 1066-1076).
[3] Campbell S, Giadresco K. Computer-assisted clinical coding: A narrative review of the literature on its benefits, limitations, implementation and impact on clinical coding professionals. Health Information Management Journal. 2020 Jan;49(1):5-18.
[4] Kelly, Liadh, et al. “Overview of the CLEF eHealth evaluation lab 2019.” International Conference of the Cross-Language Evaluation Forum for European Languages. Springer, Cham, 2019.
[5] Marimon, Montserrat, et al. “Automatic de-identification of medical texts in spanish: the meddocan track, corpus, guidelines, methods and evaluation of results.” Proceedings of the Iberian Languages Evaluation Forum (IberLEF 2019). vol. TBA, p. TBA. CEUR Workshop Proceedings (CEUR-WS. org), Bilbao, Spain (Sep 2019), TBA. 2019.
[6] Névéol, Aurélie, et al. “CLEF eHealth 2017 Multilingual Information Extraction task Overview: ICD10 Coding of Death Certificates in English and French.” CLEF (Working Notes). 2017.
[7] Névéol, Aurélie, et al. “CLEF eHealth 2018 Multilingual Information Extraction Task Overview: ICD10 Coding of Death Certificates in French, Hungarian and Italian.” CLEF (Working Notes). 2018.
[8] Pestian, John, et al. “A shared task involving multi-label classification of clinical free text.” Biological, translational, and clinical language processing. 2007.
[9] Stanfill, Mary H., et al. “A systematic literature review of automated clinical coding and classification systems.” Journal of the American Medical Informatics Association 17.6 (2010): 646-651.
[10] Aramaki E, Kano Y, Ohkuma T, Morita M. MedNLPDoc: Japanese Shared Task for Clinical NLP. InProceedings of the Clinical Natural Language Processing Workshop (ClinicalNLP) 2016 Dec (pp. 13-16).